Software Services
For Companies
For Developers
Portfolio
Build With Us
Table of Contents:
Get Senior Engineers Straight To Your Inbox
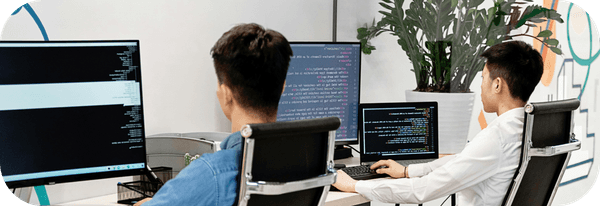
Every month we send out our top new engineers in our network who are looking for work, be the first to get informed when top engineers become available
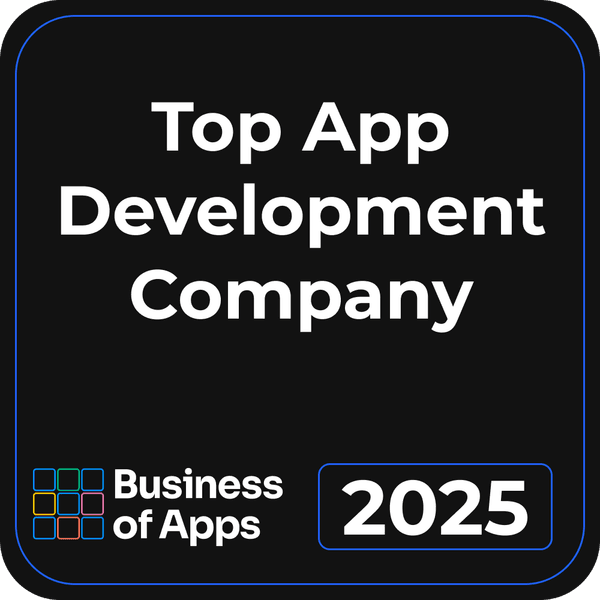
At Slashdev, we connect top-tier software engineers with innovative companies. Our network includes the most talented developers worldwide, carefully vetted to ensure exceptional quality and reliability.
Build With Us
How AI Image & Video Generation Work In 2025/

1. Introduction to AI in Visual Media/
Artificial Intelligence (AI) has revolutionized the realm of visual media, unleashing creative possibilities and redefining the way content is created, edited, and consumed. AI in visual media refers to the use of machine learning algorithms and neural networks to process, analyze, and generate visual content, encompassing everything from image and video recognition to the creation of hyper-realistic visual effects.
The integration of AI technologies in visual media allows for automated video editing, image enhancement, and content personalization. AI-driven tools can analyze vast amounts of visual data, learning from new inputs to improve their performance over time. This adaptability makes AI an indispensable asset for tasks such as:
- Automated tagging and categorization of images and videos, simplifying the management and retrieval of media assets.
- Real-time content moderation, where AI systems can quickly identify and filter out inappropriate content based on predefined criteria.
- Enhanced visual effects that would be too complex or time-consuming to create manually.
- Personalization of content in advertising and entertainment, tailoring experiences to individual preferences and behaviors.
Moreover, AI is instrumental in the development of augmented reality (AR) and virtual reality (VR) experiences, offering immersive environments that were once the stuff of science fiction. The technology’s ability to understand and interpret visual cues enables more natural and engaging interactions within these digital spaces.
For professionals and enthusiasts alike, staying abreast of AI advancements in visual media is crucial. The technology not only streamlines workflows and reduces operational costs but also opens up new avenues for artistic expression and storytelling. As AI continues to evolve, its impact on the visual media landscape promises to be both profound and transformative.
2. Evolution of AI Image Generation Technology/
The landscape of AI image generation technology has evolved at a breakneck pace, fundamentally altering the ways in which we create and interact with digital imagery. The journey from rudimentary algorithms to sophisticated neural networks marks a significant leap in AI capabilities.
Early Stages: Symbolic AI and Rule-Based Systems
In the initial stages, AI image generation relied on symbolic AI and rule-based systems. These systems operated on explicitly programmed instructions to create images based on predefined rules and logic. This method was limited in its complexity and inability to replicate the nuanced details of natural images.
The Rise of Machine Learning
As machine learning emerged, the potential for AI-generated images expanded significantly. Algorithms could now learn from a dataset of images, identifying patterns and features to replicate styles and content in new creations. This machine learning phase was marked by the use of techniques such as decision trees, support vector machines, and k-means clustering.
Neural Networks and Deep Learning
The introduction of neural networks, and more specifically deep learning, revolutionized AI image generation. Convolutional neural networks (CNNs) became instrumental in processing visual data, improving the quality and realism of generated images.
Generative Adversarial Networks (GANs)
A pivotal development in AI image generation was the advent of Generative Adversarial Networks (GANs). GANs consist of two neural networks—the generator and the discriminator—competing against each other. This results in the generation of highly realistic images, as the generator learns to produce increasingly convincing outputs to fool the discriminator.
Variational Autoencoders (VAEs)
Alongside GANs, Variational Autoencoders have played a key role in the evolution of image generation. VAEs encode input images into a lower-dimensional space and then reconstruct them, allowing for the manipulation of specific features and the creation of new images with variations of these features.
Transformer Models
The latest stride in AI image generation is the emergence of transformer models, which have initially been successful in natural language processing. These models, such as Google’s Image Transformer, are now being adapted for image generation, showing promise in capturing long-range dependencies within images.
AI Art and Style Transfer
AI’s ability to replicate artistic styles has led to the creation of ‘AI art’ through style transfer. Here, the stylistic elements of one image can be applied to the content of another, opening up new frontiers for creative expression.
The evolution of AI image generation technology continues to accelerate, with ongoing research pushing the boundaries of what’s possible. As these technologies become more sophisticated, they are enabling new applications in fields such as virtual reality, game design, and automated content creation. The future potential of AI image generation is vast, with implications for creativity, media production, and even the way we perceive art and authorship.
3. Overview of AI Video Generation: The 2025 Landscape/
AI video generation has transformed significantly by 2025, evolving from simple deepfake technologies to sophisticated systems capable of producing high-quality video content that is nearly indistinguishable from that created by humans. This technology leverages advanced machine learning algorithms, including Generative Adversarial Networks (GANs) and transformer models, to create realistic video sequences.
Key developments in AI video generation include:
- Enhanced realism: AI-generated videos now feature hyper-realistic textures, lighting, and movements, thanks to improvements in rendering algorithms and hardware acceleration.
- Customization and personalization: AI systems can generate personalized video content tailored to individual preferences, enhancing user engagement and experience.
- Real-time generation: The ability to produce videos in real-time has opened avenues for interactive applications, such as virtual reality and live-streaming events.
- Accessibility: With the democratization of AI tools, more creators have access to video generation technology, reducing the barrier to entry for high-quality video production.
- Ethical and legal frameworks: As AI-generated content becomes more prevalent, robust ethical guidelines and legal frameworks have been established to address issues of consent, copyright, and misinformation.
AI video generation’s role in various industries has expanded, influencing fields such as entertainment, marketing, education, and even healthcare. In entertainment, it is used to create stunning visual effects and resurrect historical figures in movies. Marketers leverage AI to produce dynamic advertising content that adapts to viewer responses. Educators utilize AI-generated videos to create immersive learning experiences, and healthcare professionals use it to simulate medical scenarios for training purposes.
One of the critical challenges facing this technology is ensuring authenticity and preventing misuse. Watermarking and content attribution technologies have become standard practice to verify the origin of AI-generated videos. Furthermore, public awareness and digital literacy campaigns are crucial in helping people discern AI-generated content from real footage.
As we move forward, AI video generation continues to push the boundaries of what’s possible in digital content creation. The landscape in 2025 is characterized by a blend of artistic expression and technological innovation, leading to unprecedented opportunities and challenges in the digital world.
4. The Mechanics Behind AI Image Creation/
Artificial Intelligence (AI) image creation involves a complex interplay of machine learning algorithms and neural networks that work together to generate visual content from textual descriptions or other forms of input. The process is both fascinating and intricate, relying on several key components:
-
Neural Networks: At the core of AI image generation are neural networks, particularly Generative Adversarial Networks (GANs) and Convolutional Neural Networks (CNNs). GANs consist of two parts: the generator, which creates images, and the discriminator, which evaluates them. The generator improves through feedback from the discriminator, learning to produce more realistic images over time.
-
Deep Learning: AI image creation relies on deep learning, a subset of machine learning where neural networks learn from vast amounts of data. By analyzing thousands or millions of images, the AI learns patterns and features that define various visual elements and can recreate or invent new images based on this learned information.
-
Latent Space: When discussing AI image generation, the concept of latent space is crucial. It’s a multi-dimensional space representing the features learned by the network during training. By navigating through this space, the AI can produce a wide array of images with varying features and complexities.
-
Training Data: The quality and diversity of training data are vital for the success of AI image creation. The AI needs a large dataset of images to learn from, which should be as varied as possible to allow the generation of diverse and high-quality images.
-
Optimization Techniques: To refine the image generation process, various optimization techniques are employed. These can include backpropagation and gradient descent, which help the neural networks adjust and improve through each iteration.
-
Transfer Learning: This is a method where a pre-trained neural network is fine-tuned with a smaller dataset for a specific task. In the context of image creation, it allows for the generation of images in a specific style or category without the need for extensive training from scratch.
By leveraging these mechanisms, AI is able to transform simple text prompts or other forms of input into detailed, coherent, and often stunning visual representations. The technology is rapidly evolving, pushing the boundaries of creativity and AI capabilities.
5. Deep Learning Algorithms Powering Video Synthesis/
Deep learning algorithms have revolutionized the field of video synthesis, enabling the creation of highly realistic and complex video content. These algorithms rely on neural network architectures designed to understand and replicate the intricacies of video data.
Generative Adversarial Networks (GANs) are at the forefront of video synthesis. GANs consist of two neural networks, the generator and the discriminator, which work in tandem. The generator produces video content, while the discriminator evaluates its authenticity. Through this process, GANs can generate highly realistic video clips that are often indistinguishable from real footage.
Recurrent Neural Networks (RNNs), specifically Long Short-Term Memory (LSTM) networks, are also vital in video synthesis. LSTMs are adept at handling sequential data, making them suitable for predicting future frames in a video sequence. Their ability to remember long-term dependencies allows for the creation of coherent and temporally consistent video content.
Variational Autoencoders (VAEs) offer another approach to video synthesis. By learning to encode video data into a lower-dimensional latent space and then decode it back into high-quality content, VAEs can be used to generate new videos or modify existing ones with changes in the latent space variables.
Convolutional Neural Networks (CNNs) have a different role. They are typically used for understanding and manipulating spatial information within video frames. When combined with other network types, CNNs can enhance the quality and resolution of synthesized videos, leading to more detailed and convincing results.
Transformers, originally designed for natural language processing, have recently been adapted for use in video synthesis. Their ability to handle long-range dependencies and parallelize processing makes them particularly effective for generating high-resolution videos.
To enhance the realism and dynamic nature of synthesized videos, neural rendering techniques are often employed. These techniques use deep learning to render synthetic, photorealistic images that are seamlessly integrated into video sequences. This is particularly useful for applications such as virtual reality and special effects in filmmaking.
For those looking to integrate video synthesis into their applications, it’s important to consider the computational requirements and training data needed for these algorithms. High-quality video synthesis requires significant computational power and large datasets to train the neural networks effectively.
By understanding and leveraging these deep learning algorithms, developers and content creators can push the boundaries of video synthesis, creating content that is increasingly realistic, personalized, and engaging.
6. Data Requirements for Training Image & Video Generation Models/
High-quality datasets are crucial for training image and video generation models effectively. To ensure that the models produce accurate and realistic outputs, the following data requirements should be met:
-
Volume of Data: Large quantities of images or videos are required to train a robust model. The volume of data needed can range from thousands to millions of samples, depending on the complexity of the task and the variation within the dataset.
-
Diversity of Data: The dataset should represent a wide variety of scenarios, objects, and subjects to avoid bias and overfitting. This includes different angles, lighting conditions, and backgrounds for images, as well as varied scenes and interactions for videos.
-
High-Quality Annotations: For supervised learning, each image or video in the dataset must be accompanied by accurate annotations or labels. These could be in the form of bounding boxes, object labels, or pixel-wise masks for images, and frame-wise annotations for videos.
-
Temporal Consistency in Videos: For video generation, temporal consistency is vital. The data should include sequences that allow the model to understand the continuity and flow of movement across frames.
-
Data Preprocessing: Images and videos may need preprocessing to normalize the data, such as resizing images to a standard resolution or encoding videos at a consistent frame rate.
-
Data Augmentation: To increase the robustness of the model, data augmentation techniques such as rotation, scaling, cropping, and color variation can be applied to artificially expand the dataset.
-
Ethical and Legal Considerations: It is essential to ensure that the data used for training adheres to ethical standards and complies with copyright and data privacy laws. Obtaining proper licenses and permissions for data usage is critical.
-
Data Format and Storage: Organize the dataset in a format that is accessible and efficient for training, such as using a file structure that separates data into training, validation, and test sets. Additionally, the data should be stored in a way that allows for fast reading and writing times during the training process.
Ensuring that all these requirements are met will facilitate the development of image and video generation models that are capable of producing high-quality results. Adequate attention to the diversity and quality of the training data is key to the success of these models in real-world applications.
7. Breakthroughs in Generative Adversarial Networks (GANs)/
Generative Adversarial Networks (GANs) have witnessed several groundbreaking advancements that have significantly enhanced their capabilities and applications. These breakthroughs span from improving image generation fidelity to expanding into domains like video and even 3D modeling.
-
Progress in architecture design: New GAN architectures have been introduced, such as Progressive GANs, which incrementally increase the resolution of generated images, and StyleGANs that can separate high-level attributes and stochastic variation in generated images. These innovations have allowed for unprecedented control and quality in the image generation process.
-
Training stability and efficiency: Historically, GANs have been notoriously difficult to train due to issues such as mode collapse and non-convergence. Advances in training methods have led to more stable and efficient training processes. Techniques such as spectral normalization and gradient penalty have contributed to these improvements.
-
Introduction of conditional GANs: Conditional GANs enable the generation of images conditioned on certain inputs like class labels or even text descriptions. This has opened new possibilities in personalized content creation and has significant implications for fields such as interactive design and gaming.
-
GANs in image-to-image translation: GANs have made great strides in tasks like photo-realistic image translation, enabling the conversion of images from one domain to another, such as turning sketches into realistic images or changing day scenes to night.
-
GANs for data augmentation: In contexts where data is scarce, GANs can generate additional synthetic data for training machine learning models. This has been particularly useful in fields like medical imaging where acquiring real data can be challenging or expensive.
-
Ethical considerations and countermeasures: With the improved realism of GAN-generated images and media, there has been an increased focus on the ethical implications and potential misuses, such as deepfakes. In response, there has been significant research into detection methods to differentiate between real and GAN-generated content.
-
Expansion into other domains: GANs are no longer limited to image generation; they are being used for generating music, videos, and even drug discovery. Each of these applications presents unique challenges and opportunities for further research and development.
The advancements in GAN technology are not just academic; they have real-world applications that are transforming industries. As GANs continue to evolve, we can expect them to play a pivotal role in the future of AI-driven content creation and beyond.
8. Ethical Considerations in AI-Generated Visual Content/
Understanding the ethical landscape of AI-generated visual content is crucial for creators and consumers alike. As artificial intelligence systems become more advanced and widespread, the ability to generate photorealistic images, videos, and animations raises ethical questions that must be addressed.
Transparency is key when dealing with AI-generated visual content. It’s essential to disclose the nature of the content to the audience, whether it’s for entertainment, educational purposes, or commercial use. Failing to do so can lead to misinformation, as viewers may mistake the generated content for genuine human-created material.
The potential for misuse of AI in creating deepfakes is a significant concern. Deepfakes are realistic-looking videos or images that depict people saying or doing things they never actually did. This technology can be used to create fake news, manipulate public opinion, or harm individuals’ reputations. As responsible creators, it is vital to consider the repercussions that such content can have on individuals and society.
Creators should also consider the implications of AI-generated content on intellectual property rights. As AI algorithms can produce content that closely resembles the work of human artists, it’s important to ensure that the AI creations do not infringe upon existing copyrights or trademarks.
Bias in AI is another ethical issue that cannot be ignored. AI systems are only as unbiased as the data they are trained on, and if the training data contains biases, the AI-generated visual content will likely perpetuate those biases. Creators must ensure that the datasets used to train AI algorithms are diverse and representative to avoid reinforcing stereotypes and discriminatory practices.
Finally, the use of AI in visual content creation raises questions about the future of human creativity and employment. As AI becomes more capable of performing tasks traditionally done by human artists, there is a risk that these technologies could displace creative jobs. It’s important to find a balance where AI can be used as a tool to augment human creativity, rather than replace it.
Ethical considerations in AI-generated visual content are not just an afterthought—they should be an integral part of the creation process. By addressing these concerns proactively, creators can harness the power of AI responsibly and help shape a future where technology enhances human creativity without crossing ethical boundaries.
9. Real-world Applications of AI in Image and Video Production/
Artificial Intelligence (AI) has revolutionized the field of image and video production, offering incredible advancements that were once thought to be the realm of science fiction. Here are some real-world applications of AI that are changing the industry:
-
Automated Video Editing: AI algorithms can now analyze raw footage, select the best shots, and even assemble them into a coherent narrative. This reduces the time and labor required for video editing and allows for the rapid creation of content.
-
Content Personalization: AI systems can tailor content to individual user preferences, creating a unique viewing experience. By analyzing viewing habits, AI can suggest edits or modifications that increase engagement and viewer satisfaction.
-
Visual Effects (VFX): AI has significantly enhanced the capabilities of VFX. It can automate the labor-intensive process of rotoscoping, where objects are separated from the background frame by frame. AI can also generate realistic CGI characters and environments, reducing the need for costly set constructions.
-
Image Enhancement and Restoration: AI tools can sharpen images, improve lighting, and restore old or damaged photographs and videos. This application is particularly valuable in the field of digital archiving and film restoration, where historical footage can be preserved with greater fidelity.
-
Colorization: AI can accurately colorize black and white photos and footage by learning from vast datasets of color images. This not only brings a new perspective to historical content but also aids in the educational and entertainment sectors.
-
Facial Recognition and Deepfakes: While facial recognition allows for the creation of personalized experiences and enhanced security measures, AI can also be used to create deepfakes. Deepfakes are hyper-realistic video or audio recordings that can convincingly depict events that never happened, posing ethical and legal challenges.
-
Text-to-Image Generation: AI models can generate images from textual descriptions, offering a new tool for artists and content creators to visualize concepts quickly.
-
Automated Subtitling and Closed Captioning: AI-driven speech recognition and natural language processing can automatically transcribe audio to text, making content accessible to a broader audience, including those with hearing impairments.
-
Interactive Video Content: AI facilitates the creation of interactive videos where viewers can influence the storyline or outcomes. This is particularly popular in educational content, video games, and marketing campaigns.
Each of these applications demonstrates the influence of AI on the creative process, enabling more efficient workflows, personalized content, and innovative storytelling techniques. As AI technology continues to evolve, its role in image and video production is poised to expand, leading to more sophisticated and immersive media experiences.
10. Challenges and Limitations of Current AI Visual Technologies/
Current AI visual technologies have made significant strides, but they still face several challenges and limitations that can affect their performance and applicability. Understanding these hurdles is crucial for both developers and users to set realistic expectations and work towards improvements.
-
Data Dependency: AI models require large and diverse datasets to learn effectively. However, obtaining such datasets can be difficult due to privacy concerns, intellectual property issues, and the sheer effort involved in collecting and labeling the data.
-
Bias and Ethics: AI systems can inadvertently learn and amplify biases present in their training data. This can lead to unfair or unethical outcomes, particularly in sensitive applications like facial recognition and surveillance.
-
Computational Costs: Processing and analyzing visual data is resource-intensive. The training phase for deep learning models, especially, requires significant computational power, which can be expensive and environmentally taxing.
-
Interpretability: AI decisions are often described as a ‘black box’ because it can be challenging to understand how the model arrived at a particular conclusion. The lack of transparency can be a barrier to trust and accountability, especially in critical areas such as healthcare and autonomous driving.
-
Generalization and Robustness: AI visual systems may perform well on the data they were trained on but can struggle with new, unseen scenarios. They might fail to generalize to different contexts or be easily fooled by slight alterations in the input data, known as adversarial attacks.
-
Real-time Processing: Many applications, like autonomous vehicles, require real-time analysis and decision-making. Latencies in processing can lead to outdated responses, compromising the system’s effectiveness.
-
Integration with Existing Systems: Incorporating AI into existing infrastructures can be challenging, as it often requires significant changes to accommodate the new technology.
-
Regulatory and Legal Challenges: The legal framework surrounding AI is still evolving. Issues such as data governance, privacy, and liability need to be addressed to ensure compliance and protect individuals’ rights.
-
Limitations of Current Algorithms: Despite advancements, current algorithms still have limitations in understanding context, dealing with abstract concepts, and reasoning like a human, which are essential for tasks such as natural language understanding and complex decision making.
By acknowledging and addressing these challenges, the field can continue to advance and integrate AI visual technologies more effectively into various aspects of society. Researchers and practitioners must work together to overcome these hurdles, ensuring that AI visual technologies are reliable, ethical, and beneficial for all.
11. The Future of AI in Image & Video Generation: Trends and Predictions/
Artificial Intelligence (AI) is rapidly advancing in the realm of image and video generation, leading to significant transformations in various industries ranging from entertainment to healthcare. The integration of AI with creative processes is poised to redefine the way visual content is produced and consumed.
Generative Adversarial Networks (GANs) have been at the forefront of this revolution, allowing for the creation of hyper-realistic images and videos. As the technology behind GANs improves, we can expect even more sophisticated outputs that are indistinguishable from content created by humans.
The rise of deepfake technology has also gained attention for its ability to create convincing fake videos. While this technology poses ethical challenges, it also offers exciting possibilities in areas such as film production and virtual reality. With advancements in detection algorithms, the future may see a safer application of deepfakes in controlled environments.
Customization and personalization will likely play a significant role in the future of AI-generated visual content. AI systems could tailor content to individual preferences, creating unique experiences for each user. This personalized approach could revolutionize marketing, education, and entertainment.
Increased efficiency in content production is another trend to watch. AI can significantly reduce the time and resources required to create visual content, making it accessible to a broader range of creators and businesses. This democratization of content creation could lead to a surge in innovative and diverse visual media.
Interactivity and immersive experiences are set to be enhanced by AI. As virtual and augmented reality technologies mature, AI-generated images and videos will become more interactive, providing users with immersive experiences that were previously impossible.
Ethical considerations and regulation will become crucial as AI technology becomes more prevalent in image and video generation. Ensuring responsible use and preventing misuse will require collaboration between technologists, legal experts, and policymakers.
Lastly, AI is expected to foster new art forms and creative expressions. Artists and designers are beginning to experiment with AI as a collaborative partner, leading to new aesthetics and storytelling techniques that challenge traditional boundaries.
Keeping an eye on these trends and predictions is essential for businesses, creators, and consumers alike, as they navigate the possibilities and challenges presented by the future of AI in image and video generation.
12. How to Differentiate AI-Generated Images from Authentic Ones/
Identifying AI-generated images versus authentic ones can be challenging, as technology advances, but there are key indicators that can help:
-
Look for inconsistencies: AI-generated images may have irregularities such as asymmetrical features, distorted backgrounds, or unusual patterns in textures. Authentic images are more likely to have natural imperfections and consistent lighting.
-
Check for coherence: Authentic images usually have a clear source of light and coherent shadows. In contrast, AI-generated images might display lighting and shadows that don’t align with the objects in the picture.
-
Assess the details: Pay close attention to the fine details. AI-generated images may struggle with intricate patterns, small text, or complex objects. Look for blurriness or smudging in these areas.
-
Examine facial features: If the image includes faces, scrutinize the eyes, teeth, and hair. AI often has difficulty rendering these accurately. Authentic images will generally have more naturally varied and detailed facial features.
-
Use reverse image search: If you suspect an image is AI-generated, perform a reverse image search. AI-generated images are less likely to have duplicates online, whereas authentic images may appear in different contexts.
-
Utilize detection tools: There are online tools available that can analyze images to determine if they are AI-generated. These tools look for digital fingerprints commonly left by AI image generators.
Remember, as AI technology evolves, these methods may become less effective over time. Staying informed about the latest developments in AI and image analysis can help in differentiating between AI-generated and authentic images.
13. Impact of AI-Generated Media on the Creative Industries/
AI-generated media is revolutionizing the creative industries, altering how content is produced, distributed, and consumed. As the technology behind artificial intelligence continues to advance, its impact is increasingly felt across various creative sectors, including music, literature, film, and visual arts.
In the music industry, AI algorithms can compose music that resonates with the audience’s tastes, potentially reducing the time and cost associated with creating new music. This democratization of music production allows for a surge in content creation, offering both opportunities and challenges for artists and producers. While this can lead to a more diverse range of music, it also raises concerns about originality and the devaluation of traditional composition skills.
The literature sector is witnessing the rise of AI-authored books and articles, which can draft narratives and informational content at an unprecedented pace. This technology enables publishers to meet the constant demand for new content. However, it poses questions about authorship and the future role of human writers.
In the realm of film, AI is being used to streamline the production process, from scriptwriting to visual effects and editing. This efficiency can reduce costs and allow for more experimentation, but it also brings up debates around the loss of creative jobs and the homogenization of cinematic experiences.
The visual arts have also been transformed by AI, with algorithms capable of creating artworks that mimic the styles of human artists. This can foster innovation and push the boundaries of art, but it also leads to conversations about the value of art and the definition of creativity.
For all creative sectors, one of the most significant impacts of AI-generated media is on intellectual property rights. Determining ownership and copyright for AI-generated content is complex and can lead to legal challenges. The emergence of deepfakes and other AI-manipulated media also raises ethical concerns about misinformation and the authenticity of digital content.
The creative industries must adapt to this evolving landscape, balancing the benefits of AI-generated media with the preservation of human creativity and artistic integrity. The future will likely see a hybrid approach, where AI tools augment human creativity, rather than replace it. The key will be finding ways to integrate AI that enhance the creative process while maintaining the unique value that human artists bring to the table.
14. Conclusion: The Role of AI in Shaping Visual Media/
Artificial Intelligence (AI) is revolutionizing the landscape of visual media, offering innovative tools that transform how content is created, distributed, and consumed. AI technologies are redefining the boundaries of creativity and efficiency, enabling creators to push the envelope in terms of visual effects, animation, and personalization.
The role of AI in content creation is particularly noteworthy. Through techniques like generative adversarial networks (GANs), AI can produce realistic images and videos, reducing the time and costs associated with traditional media production. AI is also enhancing the capabilities of visual effects (VFX), allowing for more complex and detailed imagery that was once thought impossible or too expensive to create.
In terms of distribution, AI algorithms are optimizing how visual media is delivered to audiences. Personalization algorithms are curating content to match viewer preferences, improving engagement, and keeping viewers hooked to platforms longer. These algorithms analyze viewing patterns to recommend content, ensuring that viewers are more likely to discover media that resonates with them.
When considering consumption, AI is changing the game through interactive media and augmented reality (AR). Viewers are no longer passive consumers; they are active participants in the visual media they engage with. AI-driven AR apps, for example, overlay digital information onto the real world, creating immersive experiences that were once the domain of science fiction.
Lastly, AI is instrumental in analyzing audience data to derive insights into viewer behavior. This data-driven approach helps creators and marketers understand what content performs well and why, leading to more effective strategies for capturing audience attention.
AI is not just a tool; it’s a transformative force that is shaping the future of visual media. Its role will continue to grow as advancements in technology create new possibilities for engagement and expression in the visual realm. The potential for AI to enhance visual media is boundless, and we are just beginning to scratch the surface of what is possible.