Software Services
For Companies
For Developers
Portfolio
Build With Us
Get Senior Engineers Straight To Your Inbox
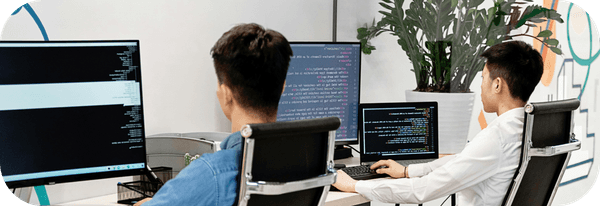
Every month we send out our top new engineers in our network who are looking for work, be the first to get informed when top engineers become available
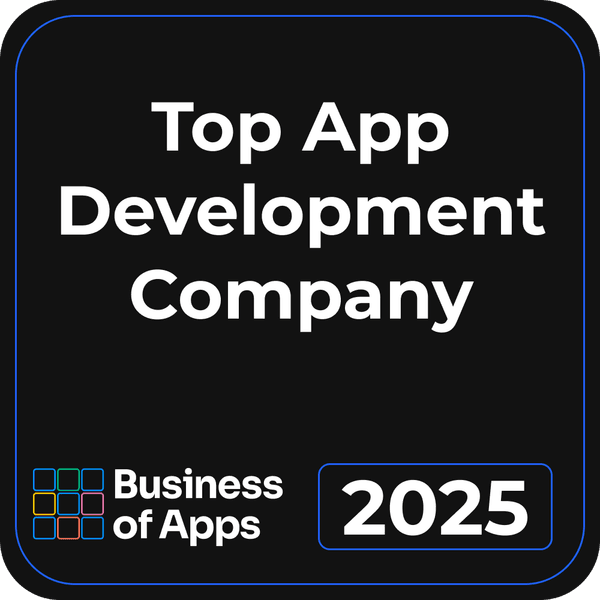
At Slashdev, we connect top-tier software engineers with innovative companies. Our network includes the most talented developers worldwide, carefully vetted to ensure exceptional quality and reliability.
Build With Us
Top 5 AI Features You Can Add to Your App with Grok 3/

Introduction to AI in App Development/
Artificial Intelligence (AI) has become a transformative force in app development, offering a myriad of possibilities for creating smarter, more intuitive applications. AI enables developers to incorporate advanced features such as machine learning, natural language processing, and predictive analytics into their apps, significantly enhancing user experience and functionality.
Machine learning algorithms are at the heart of AI integration in app development. These algorithms allow apps to learn from user interactions and improve over time, without the need for explicit programming for every scenario. For example, a music streaming app can learn from your listening habits to suggest new songs you might like, or a fitness app might adapt its workout recommendations based on your progress and feedback.
Natural language processing (NLP) is another AI technology that is increasingly being used in apps. This enables apps to understand and respond to text or voice commands in a human-like manner. Messaging apps, virtual assistants, and customer service chatbots often leverage NLP to provide a seamless and interactive user experience.
Predictive analytics is an AI feature that uses data, statistical algorithms, and machine learning techniques to identify the likelihood of future outcomes based on historical data. This can be particularly useful in e-commerce apps that predict user behavior to provide personalized product recommendations.
AI can also improve the security of apps through anomaly detection. By analyzing typical user behavior, AI can flag unusual activity that may indicate a security threat, allowing for immediate action to prevent potential breaches.
The integration of AI in app development not only enhances the capabilities of the app but also provides a competitive edge in the market. As users increasingly expect personalized and intelligent functionality, apps without AI may find it difficult to keep up.
To summarize, incorporating AI into app development is not just a trend; it’s a strategic move that can lead to more engaging, efficient, and secure applications. As AI technology continues to evolve, its role in app development will only grow more significant, paving the way for innovative applications that were once thought impossible.
Understanding Grok 3/
Grok 3 is an advanced iteration of a domain-specific language used for parsing and transforming text. This powerful tool is part of the logstash product but can be used in various other contexts where text pattern matching and filtering are essential. Understanding Grok 3 involves comprehending its basic syntax and how it can be applied to extract meaningful information from unstructured text data, such as logs or streams of text.
At its core, Grok 3 works by combining text patterns into something that matches a desired piece of text. These text patterns are called ‘grok patterns’. A grok pattern is essentially a named regular expression. By naming these patterns and combining them, developers can construct expressions that are easier to read and maintain compared to traditional regular expressions.
When working with Grok 3, the following points are crucial:
- Grok patterns are reusable. Once you’ve defined a pattern for a particular format, you can use it multiple times without having to rewrite the complex regular expression.
- Grok 3 comes with a library of predefined patterns for common data types and log formats. These can be used out-of-the-box to match IPs, usernames, numbers, and more.
- Custom patterns can be created to match specific application logs or data formats. This flexibility allows Grok 3 to be adapted to nearly any text parsing requirement.
- Efficiency in parsing can be enhanced by using only the necessary patterns and specifying the most restrictive match possible. This ensures faster processing and less resource consumption.
- The use of Grok 3 is not limited to a specific programming language or platform. It can be integrated into various data processing pipelines.
For those looking to implement Grok 3 in their systems, it’s important to start with understanding the syntax of Grok patterns. Learning how to compose a pattern from the predefined patterns and how to write custom patterns is essential. Moreover, testing Grok patterns with sample data before deployment can save a significant amount of time and prevent potential errors in data processing.
By mastering Grok 3, developers and system administrators can efficiently parse and transform logs, enabling better monitoring, searching, and analysis of data. As a result, Grok 3 becomes a powerful ally in managing and understanding the vast streams of data that modern systems generate.
AI Feature #1: Personalized Recommendations/
Personalized recommendations are a game-changer in the realm of AI applications. By leveraging user data and behavior, AI algorithms can tailor content, products, and services to match individual preferences. This personalization enhances user engagement and satisfaction, leading to increased loyalty and conversion rates.
AI-driven personalized recommendation systems operate on the principle of predictive analytics. They analyze vast amounts of data, including:
- User demographics: Age, gender, location, and more.
- Historical behavior: Past purchases, search history, and viewed items.
- Contextual information: Time of day, device used, and current trends.
The power of AI lies in its ability to learn from this data over time. As more information is gathered, the recommendations become more accurate and relevant to the user. This is done through machine learning techniques such as:
- Collaborative filtering: Suggesting items based on similar user preferences.
- Content-based filtering: Recommending items similar to what the user has liked before.
- Hybrid methods: Combining both approaches for more robust recommendations.
Personalized recommendations are not just beneficial for users; they are also invaluable for businesses. Companies that implement these systems see:
- Increased sales: By suggesting relevant products, users are more likely to make a purchase.
- Better customer retention: A personalized experience makes users feel valued, encouraging them to return.
- Higher content consumption: Personalized content feeds keep users engaged for longer periods.
To sum it up, AI-powered personalized recommendations are a cornerstone feature that significantly impacts both the user experience and business success. By delivering tailored content and suggestions, AI helps create a more intuitive and satisfying interaction between users and digital platforms.
AI Feature #2: Predictive Analytics/
Predictive analytics is a game-changer in modern technology, leveraging artificial intelligence to process vast amounts of data and forecast future events with a remarkable degree of accuracy. This capability is invaluable across myriad industries, from finance to healthcare, where making informed decisions can hinge on accurately anticipating market trends, patient outcomes, or consumer behavior.
At its core, predictive analytics involves the use of machine learning algorithms and statistical techniques to identify the likelihood of future outcomes based on historical data. It’s not about seeing into the future with a crystal ball, but rather about identifying patterns and trends that are likely to continue or emerge.
To harness predictive analytics effectively, businesses and organizations must:
- Collect and clean large datasets: The quality and quantity of data inputted into predictive models are directly proportional to the accuracy of their output.
- Choose the right algorithms and models: Different models are suited to different types of data and predictions. It’s crucial to select the one that aligns with the specific goal of the analysis.
- Continuously train and refine models: As new data comes in, models should be updated to refine their predictive capabilities.
- Implement robust data governance: Ensuring the privacy and security of data is paramount, especially with regulations like GDPR and CCPA in place.
Predictive analytics can significantly enhance decision-making processes. For example, in e-commerce, it can predict customer churn, recommend products, and optimize pricing strategies. In finance, it can assess credit risk and detect fraudulent transactions. In healthcare, it can aid in predicting patient diagnoses and outcomes, leading to better preventative care strategies.
By understanding and implementing predictive analytics, organizations can proactively address potential challenges, capitalize on emerging trends, and achieve a substantial competitive edge in their respective fields.
AI Feature #3: Natural Language Processing/
Natural Language Processing (NLP) stands as a pivotal AI feature that empowers machines to understand, interpret, and respond to human language in a valuable way. NLP is integral to creating systems that can interact with users naturally, as it involves a combination of computational linguistics—rule-based modeling of human language—with statistical, machine learning, and deep learning models.
NLP enables a variety of applications that we interact with daily, such as:
- Virtual assistants that can understand spoken commands
- Translation services that can convert text from one language to another
- Chatbots that provide customer service or support
- Sentiment analysis tools that gauge public opinion from social media content
The process of NLP involves several key tasks, including but not limited to:
- Tokenization: Breaking down text into individual words or phrases
- Part-of-speech tagging: Identifying the grammatical role of each word in a sentence
- Named entity recognition (NER): Locating and classifying entities mentioned in the text
- Dependency parsing: Analyzing the grammatical structure of a sentence
- Sentiment analysis: Determining the emotional tone behind a series of words
- Machine translation: Translating text from one language to another
Semantic analysis is a core component of NLP, which allows machines to understand the meaning and interpretation of words and sentences in context. This is complex due to the nuances of human language, including sarcasm, idioms, and varying dialects.
Developing NLP applications requires a deep understanding of both the language and the technology used to process it. Machine learning models must be trained on large datasets to effectively understand the intricacies of language. This training involves:
- Gathering and preparing a suitable dataset
- Choosing an appropriate machine learning algorithm
- Training the algorithm on the dataset
- Evaluating the model’s accuracy and refining it as needed
The future of NLP is incredibly promising, with ongoing research aiming to improve the accuracy and efficiency of language processing. As AI continues to evolve, we can expect NLP to become more sophisticated, leading to more natural and intuitive human-computer interactions.
AI Feature #4: Image Recognition/
Image recognition AI is transforming how we interact with visual data, offering remarkable capabilities for identifying and processing images. This technology relies on deep learning algorithms, particularly Convolutional Neural Networks (CNNs), to analyze visual content.
Key benefits of image recognition AI include:
- Enhanced User Experience: By automatically tagging and organizing photos, image recognition improves navigation and searchability in digital libraries.
- Security Enhancements: It’s a critical component in surveillance systems and authentication processes, such as facial recognition for unlocking devices.
- Efficiency in Healthcare: Medical diagnosis is expedited through the analysis of X-rays and MRI scans, identifying patterns that might be missed by the human eye.
- Retail and Marketing Insights: Businesses use image recognition to track inventory, analyze customer behavior, and personalize shopping experiences.
- Agricultural Optimization: Farmers can monitor crop health and detect pests or diseases early, thanks to aerial images analyzed by AI.
To leverage image recognition AI effectively, it’s essential to have a large dataset of labeled images. The AI learns from these datasets, improving its accuracy over time. Moreover, the integration of this technology must prioritize ethical considerations, such as privacy and the potential for bias in recognition systems.
For businesses looking to implement image recognition AI, it is crucial to ensure that their datasets are diverse and that the models are continuously trained to adapt to new and changing visual inputs. As the technology advances, we can expect even more innovative applications that will further integrate image recognition AI into our daily lives, making it a cornerstone of digital transformation.
AI Feature #5: Real-time User Behavior Analysis/
Real-time user behavior analysis is a transformative AI feature that significantly impacts how we understand and engage with our audience. This technology captures and interprets user actions on a website as they happen, providing immediate insights into how individuals interact with the content and features.
Benefits of real-time user behavior analysis include:
- Enhanced personalization: By monitoring user interactions live, AI can tailor content, recommendations, and offers to match user preferences, leading to a more personalized experience.
- Improved conversion rates: Understanding what users are doing in the moment allows for quick adjustments to calls-to-action and other elements to improve the chances of conversion.
- Instant feedback on changes: Implementing a new feature or design? Real-time analysis shows how users react, so you can iterate rapidly and effectively.
- Detection of unusual patterns: Spotting anomalies in behavior can reveal technical issues or highlight potential security threats like bot activity.
To leverage real-time user behavior analysis effectively, focus on the following:
- Set clear goals: Determine what specific behaviors you want to track and why. This could range from button clicks to scroll depth or time spent on a page.
- Use a robust analytics tool: Select a tool capable of handling real-time data streams and providing actionable insights.
- Integrate with other data sources: Combining real-time behavior data with historical data can enhance predictive analytics and decision-making.
- Prioritize user privacy: Ensure compliance with data protection regulations and be transparent with users about how their data is being used.
By incorporating real-time user behavior analysis, you can create a dynamic and responsive online environment that not only meets but anticipates the needs of your users, leading to a more engaging and successful online presence.
Integrating Grok 3 AI Features into Your App/
Integrating Grok 3 AI features into your application can significantly enhance user experience and functionality. Grok 3 AI offers a suite of machine learning tools that can be leveraged to provide predictive analytics, natural language processing, and pattern recognition capabilities.
To begin integrating Grok 3 AI, identify the features that best align with your app’s goals. For instance, if your app revolves around customer service, Grok 3’s natural language processing can be used to interpret and respond to customer inquiries. Alternatively, if your app deals with data analysis, predictive analytics can forecast trends and user behavior.
Ensure that your app’s infrastructure can support AI processes. Integrating AI features typically requires robust computational power and storage. Consider cloud-based solutions or upgrading your servers to meet these requirements.
Incorporate Grok 3 AI APIs to connect your app to its functionalities. These APIs serve as the bridge between Grok 3 AI and your app, allowing you to access and manipulate AI features programmatically. Make sure to follow best practices for API integration, such as using secure connections and handling exceptions properly.
Test the AI features thoroughly before deployment. It’s essential to validate that the integrated AI functions correctly and adds value to the user experience. Implement a testing protocol that includes various scenarios and user inputs to ensure reliability and performance.
Stay updated with Grok 3 AI developments. AI technology evolves rapidly, and staying informed about updates and new features can help you maintain a cutting-edge application. Implementing the latest improvements can enhance functionality and performance, keeping your app relevant and competitive.
By carefully selecting, integrating, and maintaining Grok 3 AI features in your app, you can offer users a more personalized and efficient experience, setting your application apart in a competitive market.
Best Practices for Implementing AI with Grok 3/
Understand Grok 3 Architecture: Before implementing AI with Grok 3, it’s essential to have a solid understanding of its architecture. Knowing how the system processes data and performs tasks allows you to align your AI models and workflows with the platform’s strengths.
Data Preparation: Proper data preparation is crucial for the success of AI projects. Ensure that your datasets are clean, well-organized, and annotated when necessary. Grok 3 can process a variety of data formats, but optimizing your data structure for easy ingestion will streamline operations.
Model Training: When training AI models with Grok 3, use a diverse and representative dataset to avoid bias and ensure that the model performs well across different scenarios. Monitor the training process to fine-tune hyperparameters and prevent issues such as overfitting.
Integration: AI models need to be integrated seamlessly into existing systems. With Grok 3, ensure that your AI models communicate efficiently with other components and services within the ecosystem. Proper integration leads to more robust and scalable AI solutions.
Testing and Validation: Rigorous testing is necessary to gauge the effectiveness of your AI implementation. Use a variety of test cases to cover all possible use scenarios. Validation helps in confirming that the AI model meets the required performance benchmarks before deployment.
Monitoring and Maintenance: Post-deployment, continuous monitoring is imperative to track the performance of your AI models. Grok 3 may offer tools to assist in this process. Regular maintenance and updates will help in adapting to new data and evolving use cases.
Compliance and Security: Ensure your AI implementation complies with relevant regulations and standards. Grok 3 should be configured to maintain high levels of security, protecting sensitive data and AI models from unauthorized access.
Scalability: Design your AI solutions with scalability in mind. Grok 3 should handle increasing workloads and expanding datasets without significant drops in performance. Plan for future expansion to accommodate growth.
Documentation and Knowledge Sharing: Maintain detailed documentation of the AI implementation process. This facilitates troubleshooting, future modifications, and knowledge transfer among team members.
Leverage Community and Support: Utilize the community around Grok 3 and official support channels for guidance and best practices sharing. Engaging with the community can provide insights into optimizing your AI implementation.
By adhering to these best practices, you can harness the full potential of AI with Grok 3, ensuring that your projects are not only technically sound but also poised for success in both the short and long term.
Security Considerations for AI Features in Apps/
When integrating AI features into your applications, security should be a top priority. The complexity and sophistication of AI systems can introduce unique security challenges that must be addressed to protect user data and maintain trust.
Ensure Data Privacy: Any AI system that processes personal data must comply with relevant privacy regulations such as GDPR or CCPA. This involves obtaining explicit consent from users, providing clear data usage policies, and implementing features that allow users to view, edit, or delete their personal information.
Protect Data with Encryption: Data both at rest and in transit should be encrypted. Use strong encryption protocols to safeguard sensitive user data from unauthorized access. This is particularly important for applications that utilize cloud-based AI services.
Regularly Update AI Models: AI models can become outdated, leading to vulnerabilities. Regular updates and patches are essential to address any security flaws and improve the models’ accuracy and efficiency.
Control Access with Authentication: Implement robust authentication mechanisms to control access to AI features. Multi-factor authentication (MFA) can significantly reduce the risk of unauthorized access.
Monitor for Adversarial Attacks: AI systems are susceptible to adversarial attacks, where inputs are manipulated to confuse the model. Continuous monitoring for unusual patterns and inputs can help detect and mitigate these attacks.
Secure APIs: If your application relies on APIs to access AI services, ensure those APIs have proper security measures in place, such as rate limiting and access controls.
Conduct Regular Security Audits: Regular security audits can help identify vulnerabilities within your AI implementation. This should involve both automated tools and manual review by security experts.
Implement Responsible AI Practices: Foster an ethical AI environment by ensuring that the AI systems do not exhibit bias and that decisions made by AI are explainable and transparent.
By addressing these security considerations, developers can create AI features in apps that are not only innovative and powerful but also secure and reliable for users.
Measuring the Impact of AI Features on User Engagement/
Understanding user engagement is critical when measuring the impact of AI features on a platform. User engagement metrics provide insights into how users interact with the AI functionalities and help in assessing their value.
Key user engagement metrics to track include:
- Time on Page: This indicates how long users stay on pages that contain AI features. An increase in time spent might suggest that users find the AI elements engaging.
- Bounce Rate: The rate at which users leave after viewing only one page. A lower bounce rate on AI-driven pages may point to a positive user experience.
- User Retention Rate: Measures how often users return to use the AI functionality. High retention rates can signal a successful integration of AI into the user experience.
- Conversion Rate: The percentage of users who take a desired action, like signing up or making a purchase, after interacting with AI features. A higher conversion rate can imply that AI is effectively driving users towards business goals.
- Click-Through Rate (CTR): For AI features that suggest content or products, the CTR can indicate the relevance and success of these recommendations.
Qualitative feedback is also invaluable. Surveys, user interviews, and usability tests can reveal how users perceive the AI features and what improvements are necessary.
A/B testing is a powerful method for directly measuring the impact of AI. By presenting a version of your platform with AI features to one group of users and a version without to another, you can compare engagement data to see the difference AI makes.
Heatmaps and session recordings can visually demonstrate how users interact with AI elements on a page. These tools can uncover patterns that numbers alone might not reveal, such as hesitation or confusion in user interactions.
It’s essential to correlate user engagement data with AI performance metrics. For instance, if an AI recommendation engine is being used, you should track the accuracy and relevance of the recommendations alongside user engagement metrics to see if there is a direct relationship.
Regular monitoring and analysis of these metrics are necessary to optimize AI features and ensure they continue to enhance user engagement over time. By effectively measuring the impact of AI, businesses can make informed decisions to improve user experience and achieve their strategic objectives.
Conclusion: Future-Proofing Your App with AI/
Integrating AI into your application is not just a trend, but a strategic move to future-proof your business. As technology evolves, AI becomes increasingly crucial in providing cutting-edge solutions that keep your app relevant and competitive.
To future-proof your application with AI, consider the following steps:
- Embrace AI-driven analytics to gain insights into user behavior, enabling personalized experiences and better decision-making.
- Invest in machine learning algorithms that can adapt over time, improving the functionality of your app as more data becomes available.
- Automate routine tasks with AI to enhance efficiency and allow your team to focus on more creative and strategic endeavors.
- Ensure your AI systems are scalable so they can grow with your user base and the complexity of their needs.
- Stay informed about AI advancements and be prepared to integrate new features and technologies that can benefit your app.
- Prioritize data privacy and security in all AI implementations to maintain user trust and comply with regulations.
Remember, the integration of AI should not be an afterthought but a core consideration in the design and development of your application. By keeping these principles in mind, you can create an app that not only meets the current demands but is also ready for the challenges and opportunities that the future may bring.