Software Services
For Companies
Portfolio
Build With Us
Table of Contents:
Harnessing the Potential of Machine Learning in Financial Services: Innovations and Opportunities/

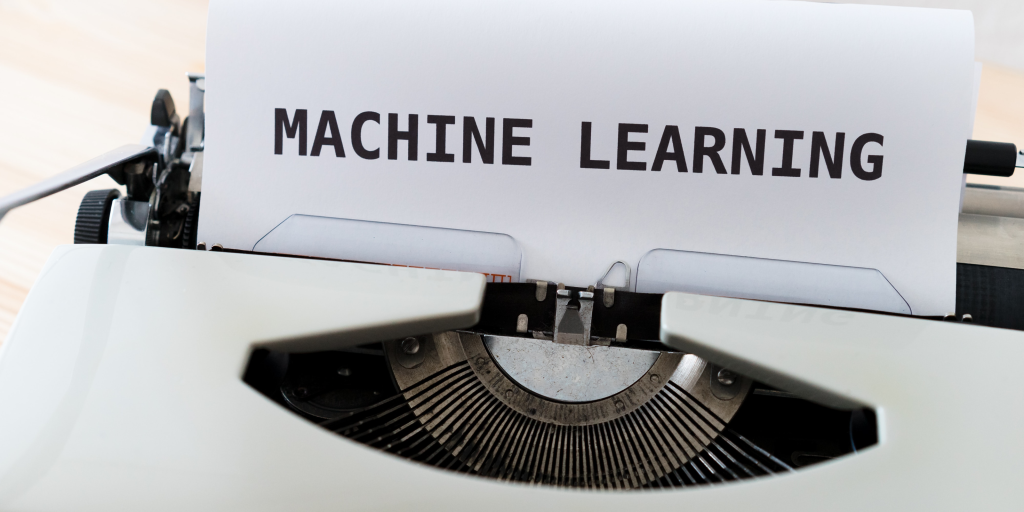
Introduction
Machine learning has emerged as a powerful tool in transforming the financial services industry. With its ability to analyze vast amounts of data and uncover hidden patterns, machine learning is revolutionizing various aspects of finance, from fraud detection and risk assessment to customer experience and investment strategies. In this article, we will explore the potential of machine learning in financial services, the innovations it has brought, and the opportunities it presents.
Machine Learning in Financial Services
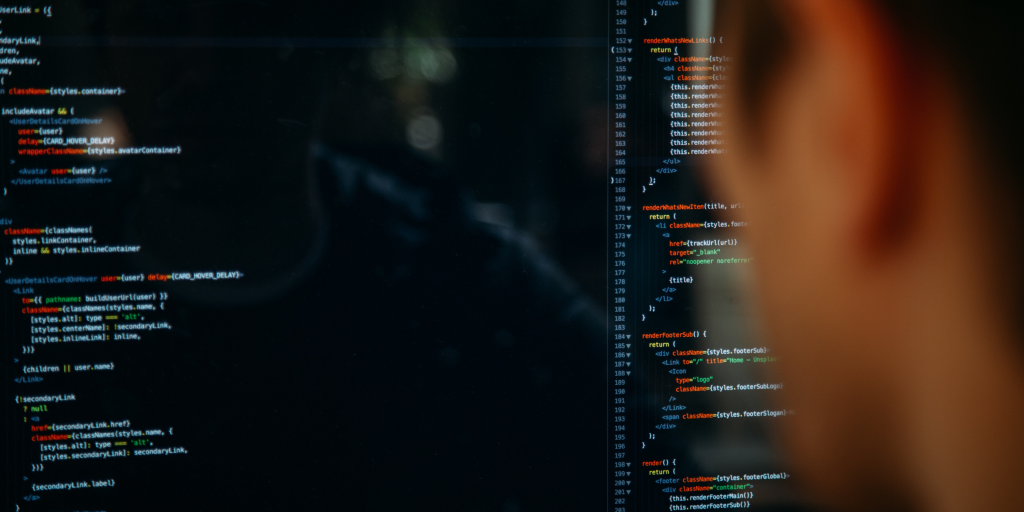
Fraud Detection and Prevention
Machine learning algorithms can analyze large volumes of financial data to detect fraudulent activities in real time. By identifying patterns and anomalies, machine learning models enhance fraud prevention measures and reduce financial losses for both financial institutions and customers.
Risk Assessment and Credit Scoring
Machine learning algorithms can assess creditworthiness and predict default risks by analyzing historical data, transaction patterns, and credit history. This enables more accurate credit scoring, improved lending decisions, and better risk management.
Algorithmic Trading and Investment Strategies
Machine learning algorithms enable automated trading by analyzing market data, identifying trends, and executing trades in real time. These algorithms can also optimize investment strategies by predicting market movements and identifying opportunities for portfolio diversification.
Innovations in Financial Services
Chatbots and Virtual Assistants
Machine learning-powered chatbots and virtual assistants are transforming customer interactions in the financial industry. These intelligent systems can provide personalized assistance, answer queries, and even perform simple transactions, enhancing customer experience and efficiency.
Robo-advisors
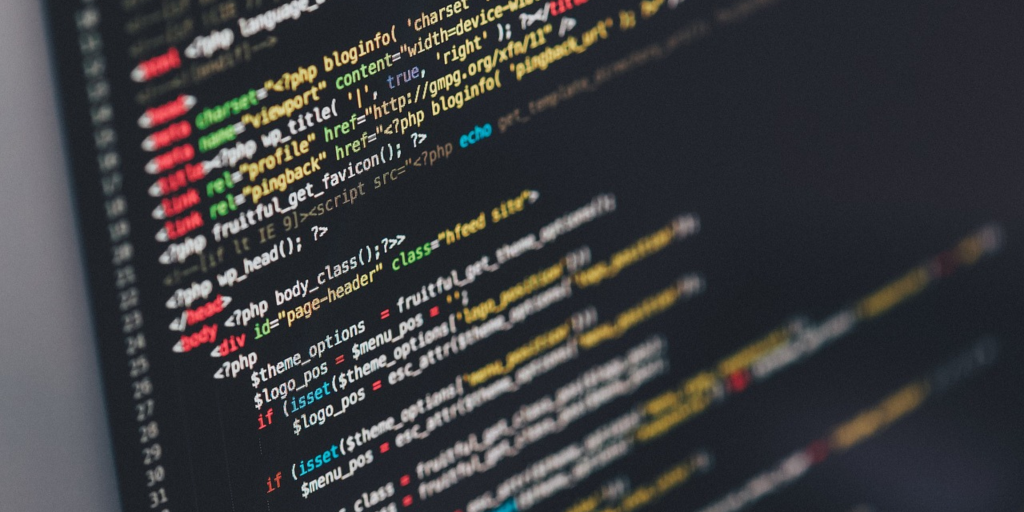
Robo-advisory platforms leverage machine learning algorithms to provide automated investment advice and portfolio management services. By analyzing financial goals, risk tolerance, and market conditions, robo-advisors offer personalized investment recommendations at a fraction of the cost of traditional financial advisors.
Natural Language Processing (NLP) for Sentiment Analysis
NLP techniques combined with machine learning algorithms can analyze customer feedback, social media data, and news sentiment to gauge market trends, customer satisfaction levels, and brand reputation. This information can inform business strategies and improve decision-making processes.
Overcoming Challenges and Ensuring Ethical Use
Data Privacy and Security
The use of machine learning in financial services raises concerns about data privacy and security. It is crucial for organizations to implement robust data protection measures, adhere to regulatory requirements, and ensure the ethical use of customer data.
Explainability and Transparency
Machine learning models often operate as “black boxes,” making it challenging to understand how they arrive at their predictions. It is essential to develop interpretable and explainable machine learning models, especially in high-stakes financial applications, to ensure transparency and build trust.
Bias and Fairness
Machine learning algorithms are susceptible to bias if the training data reflects existing societal biases. Financial institutions must actively address bias and fairness concerns by using diverse and representative datasets, regularly monitoring algorithm performance, and implementing safeguards to mitigate bias.
Opportunities for Collaboration and Innovation
Partnerships with Fintech Startups
Financial institutions can collaborate with fintech startups specializing in machine learning to leverage their expertise and innovative solutions. This collaboration can drive digital transformation, enhance operational efficiency, and deliver new and improved financial services.
Talent Acquisition and Upskilling
To harness the potential of machine learning in financial services, organizations must invest in talent acquisition and upskilling programs. Hiring data scientists and machine learning experts, as well as providing training to existing staff can build a skilled workforce capable of leveraging machine learning effectively.
Conclusion
Machine learning is reshaping the financial services landscape, enabling organizations to make data-driven decisions, improve customer experiences, and mitigate risks. By embracing machine learning, financial institutions can unlock new opportunities, enhance operational efficiency, and stay ahead in an increasingly competitive industry.
To explore the possibilities of machine learning in financial services and stay updated with the latest trends and innovations, visit slashdev.io. Access valuable resources, articles, and community discussions that delve deeper into machine learning applications in finance.
Embrace the power of machine learning and transform your financial services offerings. Embrace innovation, harness the potential of data, and embark on a journey of intelligent finance with machine learning as your guide. The future of financial services is here, and it’s driven by machine learning.